Active Projects
Dual-hand Mirroring Exoskeleton to Regain Hand Function Post-Stroke
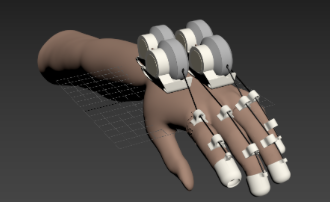
Project Members:
Anida Len, Brittany Powell, Shuyang Zhou, Hannah Stuart
Data-driven discovery of biomechanical biomarkers of chronic low back pain
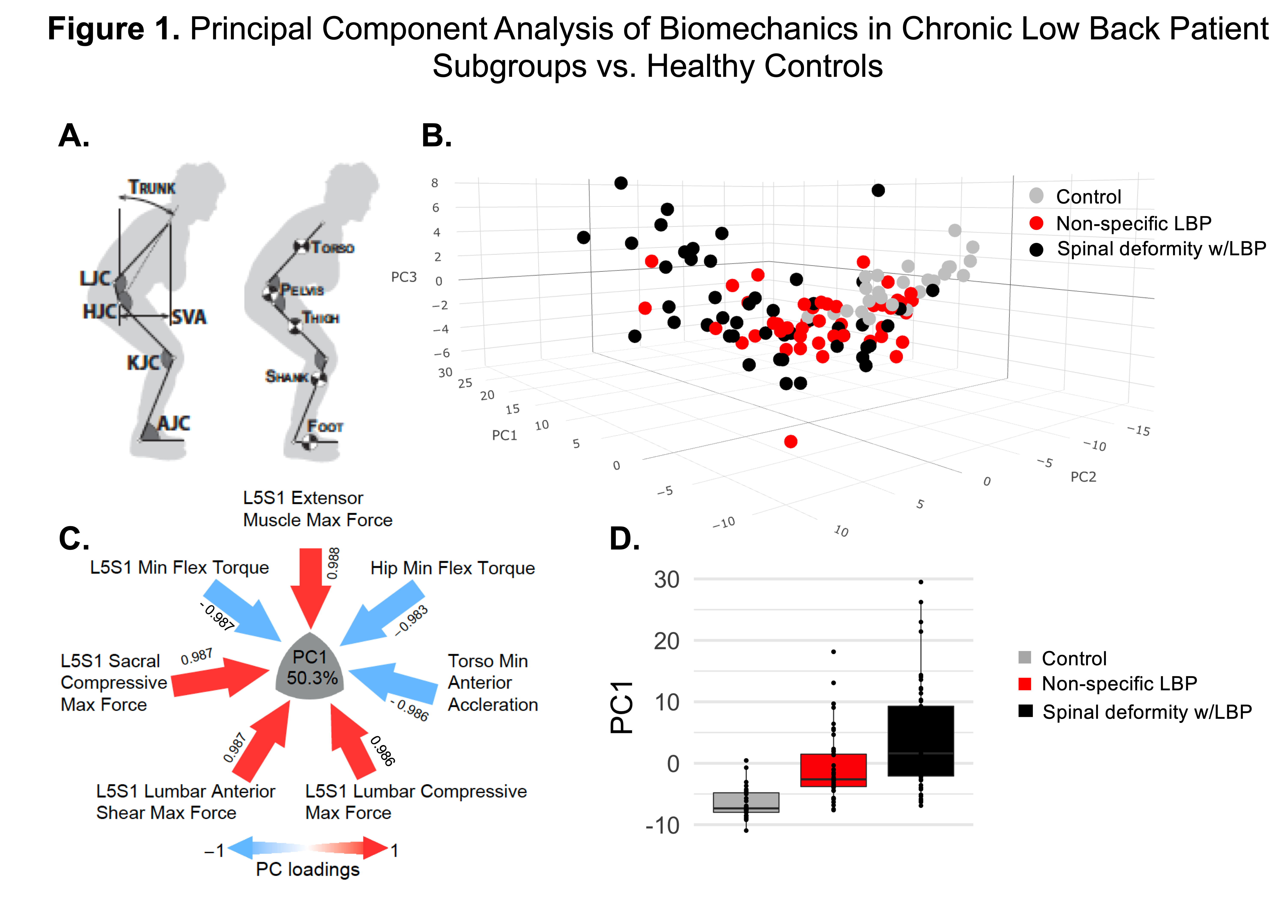
Project Members:
Anastasia Keller, Jeannie Bailey, Abel Torres Espin, Adam Ferguson
As a leading cause of disability worldwide, chronic low back pain (cLBP) represents a significant medical and socioeconomic problem with estimated health care spending of $87 billion/year. The lack of clinical tests to identify relevant biopsychosocial processes responsible for individual experience of cLBP has resulted in overall poor patient outcomes, despite substantial research efforts on mechanisms and management of cLBP. Severeal research studies have demonstrated the potential utility of kinematic measures to facilitate cLBP patient functional assessment, however, there is still a lack of consensus about which biomechanical outcomes should be measured. The UCSF REACH Physical Function and Biomechanics core (led by Dr. Bailey & Dr. Matthew) developed and validated 3D depth mapping sensor technology generating accurate measures of spine, hip and knee joint-positions, sagittal vertical axis, forces and muscular moments at multiple joints. Using tools from the Ferguson lab, we performed proof-of-concept principal component analysis (PCA) on a convenience sample of subjects assessed using sit-to-stand (schematically shown in Fig. 1A) with over 100 biomechanical variables collected. The preliminary results demonstrate the sensitivity of these biomechanical metrics to discriminate between 3 groups of subjects: healthy controls (n=26), spinal deformity patients with cLBP indicated for surgery (n= 46) and patients with non-specific cLBP (n=45) (Fig.1 B, D). Based on just the top 6 loadings of the principal component 1 (PC1) (Fig. 3C), the key separating features between patients with cLBP and healthy controls include lumbar and sacral spine forces (higher in patient groups), spine, hip torques and torso acceleration (reduced in patients with cLBP). Importantly, the biomechanic variables have high sensitivity to separate between the two patient groups: non-specific cLBP and adult spinal deformity patients with cLBP who have significantly higher pain scores (data not shown), suggesting that the impairment in movement is dependent on pain severity. The analysis is still ongoing with our long-term objective to identify a sensitive biomechancal biomarker that could be translated into clinically useful diagnostic tool which will facilitate clinical decision making regarding therapeutic management on case-by-case basis to promote compliance with the overall objective for precision-based, patient-centric medical care.
Quantifying and Predicting Risk of Fracture and Implant Failure in Individuals with Transfemoral Osseointegrated Prostheses
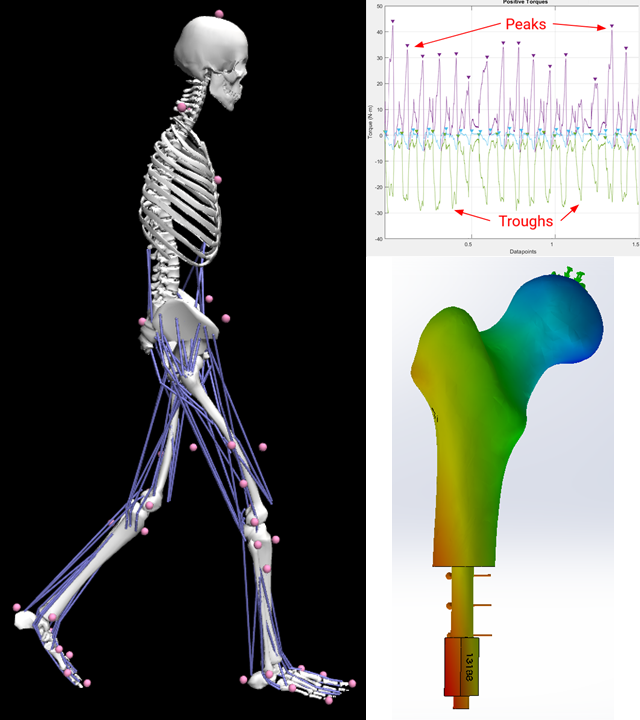
Project Members:
Zachary Lima, Dowell Mbanu-Jackson, Grace O'Connell
Former Members:
Sami Lama, Himani Patel
There are approximately 1.6 million individuals with lower limb amputation in the United States, with an associated healthcare cost of $8.3 billion. This number is expected to double by 2050, primarily due to vascular diseases such as diabetes. For amputees there are two types of methods for attaching prosthetic limbs to lower body amputations: conventional socket-type prosthetics and osseointegrated prosthetics.
Conventional socket-type prosthetics possess a myriad of deficiencies, including poor ventilation and inadequate distribution of the high forces experienced by the residual limb. Roughly 40% of lower limb amputees will suffer from skin breakdown while 30% have long term pain. Alternatively, the residual bone can be connected to the external prosthesis using an osseointegrated interface, thus eliminating the need for a socket. As a result, osseointegration devices can be used instead of socket-type prosthetics to provide decreased pain, increased proprioceptive feedback, and improved patient-reported-outcomes.
Working alongside the UCSF departments of Physical Therapy and Rehabilitation Science, Orthopedic Surgery, as well as the UCSF METRiCS research group, the capstone team led by Dr. Robert Matthew and Dr. Grace O’Connell work to analyze data from Vicon motion sensors and iPecs force sensors used by individuals with transfemoral osseointegrated prostheses from multiple physical activity tests. Data is analyzed using MATLAB and OpenSim software to characterize loading conditions used for Finite Element Analysis (FEA) simulations in Solidworks. In addition, the team will focus on developing a realistic FEA simulation that incorporates different bone densities that mimic various bone density regions that are caused by stress shielding. Using the data produced and the simulation results, the team can characterize how the human body and implant react to the various biomechanical loads produced from different physical activities.
Former Projects
Star Excursion Balance Test
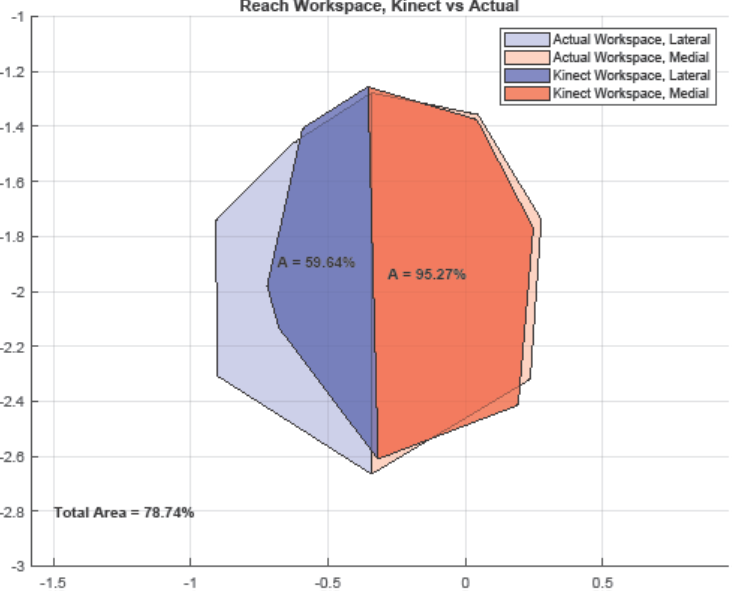
Project Members:
Ryan Halvorson, Francine Castillo, Karim Khattab, Brian Feeley
Postural control is the ability to maintain body position and control body movements. Dynamic postural control involves the ability to maintain center of mass within the base of support during various movements. The Star Excursion Balance Test (SEBT) is a popular method of measuring dynamic postural control and core stability. However, the original SEBT has several limitations— it is difficult to administer manually and has limited interobserver reliability, and it fails to quantify other potentially valuable information about joint kinematics and postural control during the test. This project aims to use the Microsoft Kinect camera to 1. Quantify the results of the original SEBT and 2. Augment the original SEBT with additional metrics such as functional workspaces, joint kinematic, and dynamics. Pilot data suggests that using the Kinect is a reliable and effective method of administering the SEBT. Future studies will involve longitudinal collection of data to assess the relationship between SEBT performance and injury risk.
Automated Classification of Spine, Hip, and Knee Pathologies from Sit-to-Stand Movement Using Depth Cameras and Machine Learning
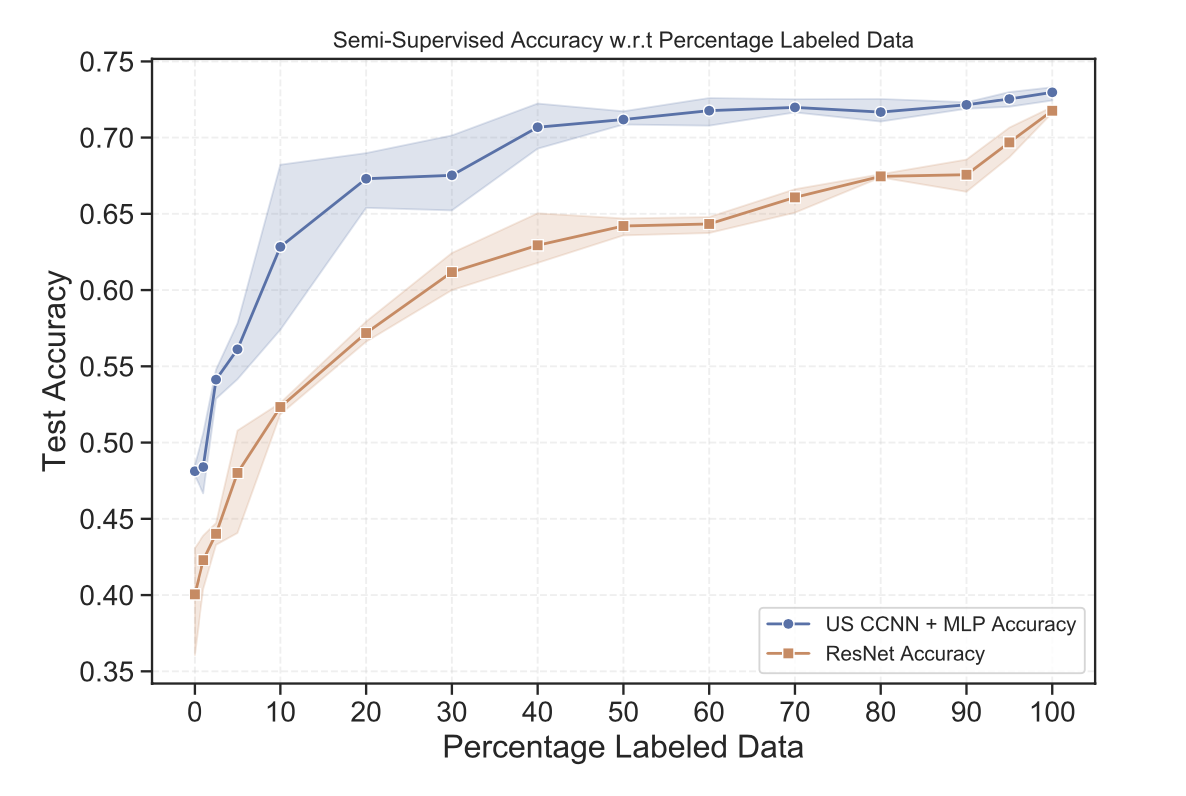
Project Members:
Harshayu Girase
Efficient, cost-effective methods for point-of-care quantification of patient biomechanics can facilitate faster and more accurate diagnoses. In this work, we present a new method to diagnose pre-surgical back, hip, and knee patients by analyzing their sit-to-stand motion captured by a Kinect camera. From patients’ motion capture data, we extract biomechanical (kinematic, kinetic, and dynamic) time-series features and provide a comprehensive assessment on the use of machine learning for patient classification. We compare the performance of models trained on time-series data to models trained on extracted scalar features, and show the importance of exploiting full time-series data for this specific classification task. Additionally, we demonstrate the effectiveness of semi-supervised learning in problems with limited and noisy labelling. Our best semi- supervised model achieves ∼ 73% accuracy in distinguishing pre- surgical back, hip, and knee patients from control subjects – a non-trivial task when only considering the sit-to-stand motion.
Publications:
Girase, H., Nyayapati, P., Booker, J., Lotz, J. C., Bailey, J. F., & Matthew, R. P. (2021). Automated assessment and classification of spine, hip, and knee pathologies from sit-to-stand movements collected in clinical practice. Journal of Biomechanics, 128, 110786 (link)